
HM01B0 QVGA Monochrome DVP Camera Module for Arduino GIGA R1 WiFi Board
Low light sensitive, ultralow power consumption, simple interface, compatibility with Arduino libraries, suitable for battery-powered and energy-efficient applications.
Overview
This camera is based on HM01B0 QVGA monochrome rolling shutter image sensor. The sensor is an Ultra Low Power Image Sensor designed for Always-on vision devices and applications. With high light sensitivity and on-chip self oscillator, you can get better image quality under diifferent light conditions.
The low power consumption camera module is equipped with a 60°(D) stock Lens. It supports RAW6/8 output format. The standrad operating temperature is between -30~85℃, with the stable Image temp. between 0~50℃.
It is fully compatible with Arduino GIGA R1 WiFi board, a professional-grade microcontroller development board recently launched by Arduino. Seamlessly integrates with our camera module, unlocking versatile image capture and processing capabilities. Harness the power of Arduino GIGA R1 WiFi and gain a competitive edge in your projects.
Features:
- Cost-Effective: Affordable solution for implementing visual capabilities in cost-sensitive projects.
- Compact Size: Compact form factor enables integration into space-constrained applications.
- Simplify Embedded Vision for All: Easy to set up and operate. Making it possible to add machine vision to your GIGA R1 board without much effort at all.
- High Sensitivity: High low-light sensitivity 3.6µ BrightSenseTM pixel technology. With on-chip self oscillator.
- Ultralow Power Consumption: Efficient power usage, making it suitable for battery-powered and energy-efficient applications.
Tech specs
Image Sensor | HM01B0 |
Resolution | QVGA |
Optical Size | 1/11” |
Number of effective pixels | 320(H) × 320(V) |
Pixel Size | 3.6μm × 3.6μm |
Support Platform | Arduino GIGA R1 WiFi Board |
Shutter Type | Electronic Rolling Shutter |
Color Filter Array | Monochrome |
Frame Rate | 320×240/160×120@30fps |
Output Format | RAW8 |
F.NO | 2.8±5% |
Focus Type | Fixed Focus |
Focal Length | 1.47mm |
Field of View(FOV) | 60°(D) |
Power Supply | AVDD: 2.8V; DOVDD: 1.8V; DVDD: 1.5V |
Operating Temperature | -30~85℃ |
Camera Board Size | 30.5mm x 30.5mm |
Documentation
Get Inspired
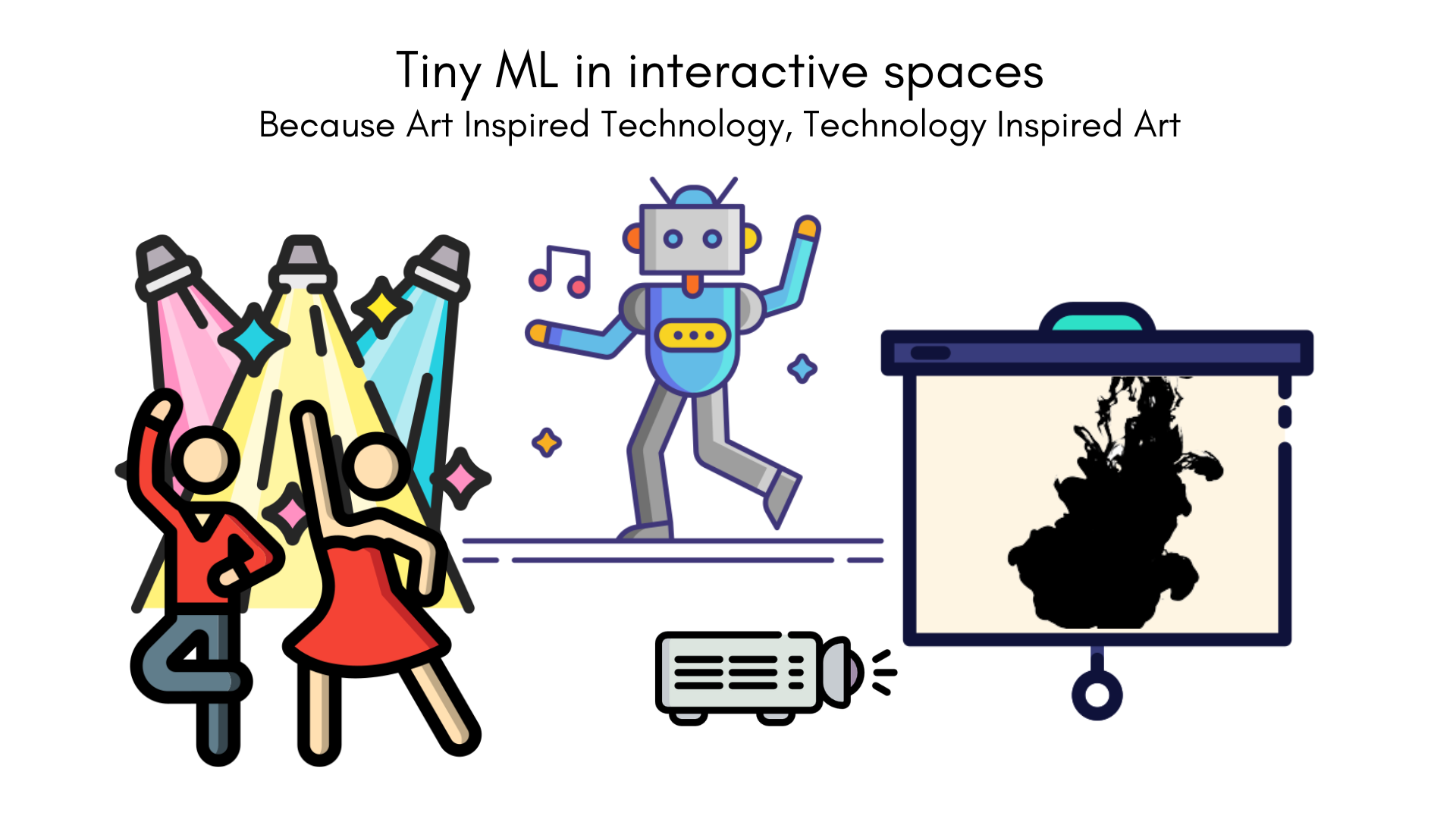
An intelligent device to track moves with responses during an interactive space with mapping, backlight, music and smart sculptures. This project makes use of a machine learning algorithm capable of tracking and detecting moves to identify associated gesture recognition through a microcontroller. Smart sculptures, lighting, music and video projection to trigger with each assigned gesture, creating a powerful AV experience highlighting the incredible potential of TinyML for the performing arts. This allows the corresponding media set Tiny ML in interactive to play when the right move was made because all these elements interact to create a new experience. This allows us to create Interactive installations, these sculptures use a combination of motors, sensors, and other electronics to create an immersive and interactive experience for the viewer. They may include projections, sound, and other sensory elements to create a complete experience.
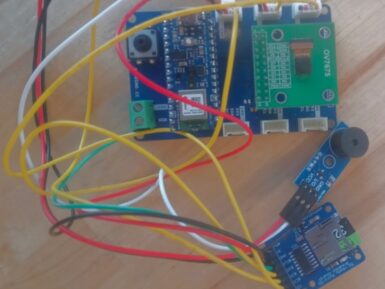
With an array of onboard sensors, Bluetooth® Low Energy connectivity, and the ability to perform edge AI tasks thanks to its nRF52840 SoC, the Arduino Nano 33 BLE Sense is a great choice for a wide variety of embedded applications. Further demonstrating this point, a group of students from the Introduction to Embedded Deep Learning course at Carnegie Mellon University have published the culmination of their studies through 10 excellent projects that each use the Tiny Machine Learning Kit and Edge Impulse ML platform. Wrist-based human activity recognition Traditional human activity tracking has relied on the use of smartwatches and phones to recognize certain exercises based on IMU data. However, few have achieved both continuous and low-power operation, which is why Omkar Savkur, Nicholas Toldalagi, and Kevin Xie explored training an embedded model on combined accelerometer and microphone data to distinguish between handwashing, brushing one’s teeth, and idling. Their project continuously runs inferencing on incoming data and then displays the action on both a screen and via two LEDs. Categorizing trash with sound In some circumstances, such as smart cities or home recycling, knowing what types of materials are being thrown away can provide a valuable datapoint for waste management systems. Students Jacky Wang and Gordonson Yan created their project, called SBTrashCat, to recognize trash types by the sounds they make when being thrown into a bin. Currently, the model can three different kinds, along with background noise and human voices to eliminate false positives. Distributed edge machine learning The abundance of Internet of Things (IoT) devices has meant an explosion of computational power and the amount of data needing to be processed before it can become useful. Because a single low-cost edge device does not possess enough power on its own for some tasks, Jong-Ik Park, Chad Taylor, and Anudeep Bolimera have designed a system where