
Overview
The MKR THERM Shield allows a board of the MKR family to acquire temperatures from a thermocouple of type K and a DS18Bxx digital one wire sensor. The Thermocouple is a kind of temperature sensor that offers high accuracy and a wide range of measured temperatures. Its analog nature requires specific interfacing and this shield relies on the MAX31855 thermocouple digital interface.
The two connectors of the MKR THERM Shield allow the usage of Type K thermocouples with or without a plug attached to the metal wires.
The MKR THERM Shield has its own library to read the temperature measured by the thermocouple. You may find further details and a usage example in our Getting Started page.
Tech specs
Connectors |
K TYPE DALLAS DS18S20 (to solder) Screw terminal block |
Input Voltage | 3.3V |
Operating Voltage | 3.3V |
Operating Temperature | -200°C + 700°C |
Communication | SPI/1Wire |
IC | MAX31855 |
Length | 61 mm |
Width | 25 mm |
Weight | 32 gr. |
Conformities
Resources for Safety and Products
Manufacturer Information
The production information includes the address and related details of the product manufacturer.
Arduino S.r.l.
Via Andrea Appiani, 25
Monza, MB, IT, 20900
https://www.arduino.cc/
Responsible Person in the EU
An EU-based economic operator who ensures the product's compliance with the required regulations.
Arduino S.r.l.
Via Andrea Appiani, 25
Monza, MB, IT, 20900
Phone: +39 0113157477
Email: support@arduino.cc
Documentation
OSH: Schematics
The Arduino MKR Therm Sheld is open-source hardware! You can build your own board using the following files:
EAGLE FILES IN .ZIP SCHEMATICS IN .PDFLearn more
Get Inspired
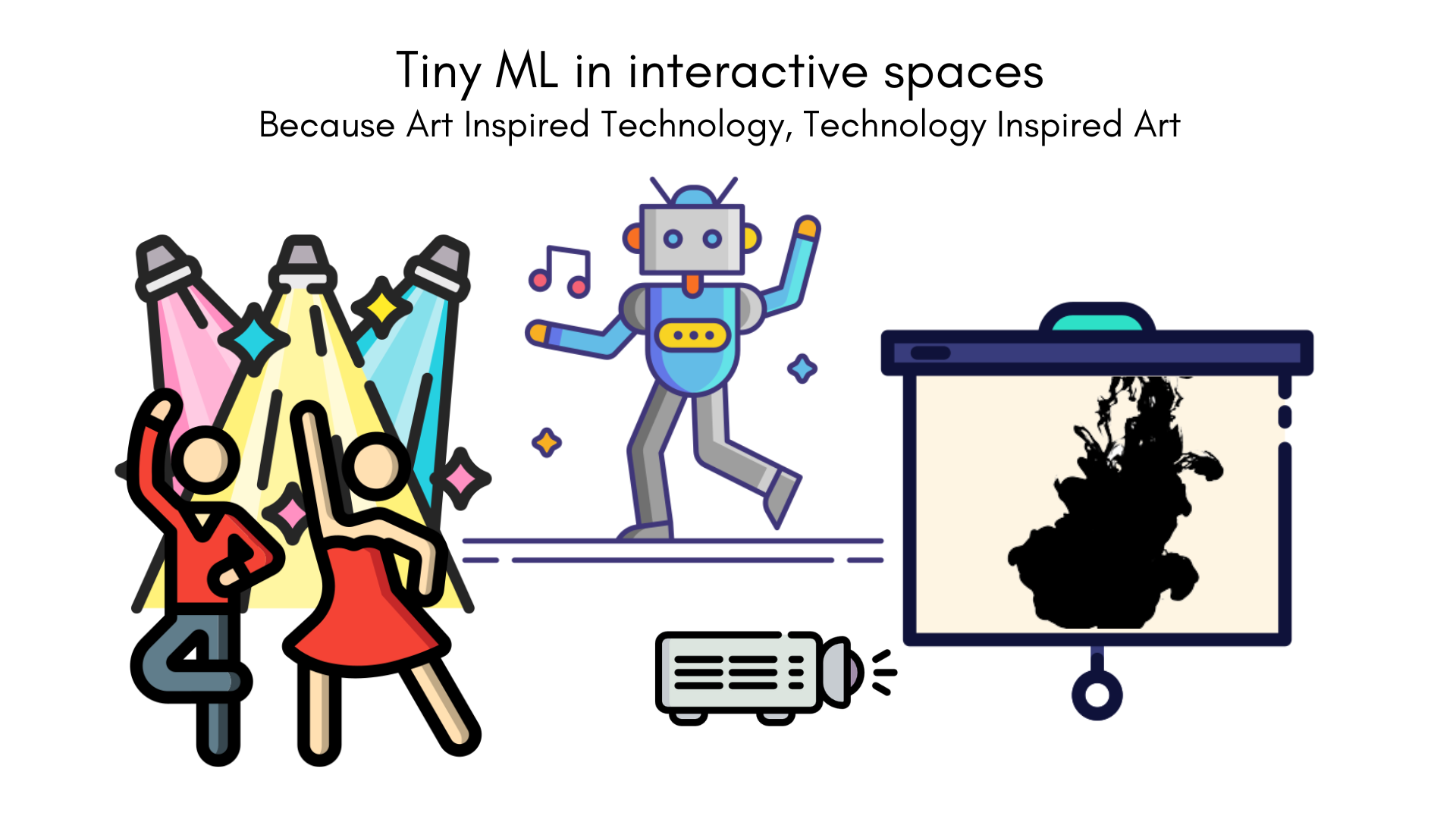
An intelligent device to track moves with responses during an interactive space with mapping, backlight, music and smart sculptures. This project makes use of a machine learning algorithm capable of tracking and detecting moves to identify associated gesture recognition through a microcontroller. Smart sculptures, lighting, music and video projection to trigger with each assigned gesture, creating a powerful AV experience highlighting the incredible potential of TinyML for the performing arts. This allows the corresponding media set Tiny ML in interactive to play when the right move was made because all these elements interact to create a new experience. This allows us to create Interactive installations, these sculptures use a combination of motors, sensors, and other electronics to create an immersive and interactive experience for the viewer. They may include projections, sound, and other sensory elements to create a complete experience.
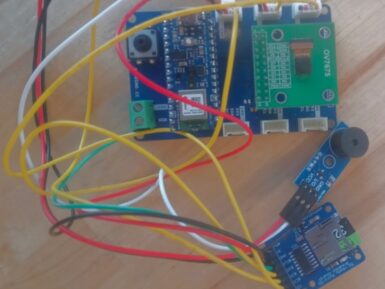
With an array of onboard sensors, Bluetooth® Low Energy connectivity, and the ability to perform edge AI tasks thanks to its nRF52840 SoC, the Arduino Nano 33 BLE Sense is a great choice for a wide variety of embedded applications. Further demonstrating this point, a group of students from the Introduction to Embedded Deep Learning course at Carnegie Mellon University have published the culmination of their studies through 10 excellent projects that each use the Tiny Machine Learning Kit and Edge Impulse ML platform. Wrist-based human activity recognition Traditional human activity tracking has relied on the use of smartwatches and phones to recognize certain exercises based on IMU data. However, few have achieved both continuous and low-power operation, which is why Omkar Savkur, Nicholas Toldalagi, and Kevin Xie explored training an embedded model on combined accelerometer and microphone data to distinguish between handwashing, brushing one’s teeth, and idling. Their project continuously runs inferencing on incoming data and then displays the action on both a screen and via two LEDs. Categorizing trash with sound In some circumstances, such as smart cities or home recycling, knowing what types of materials are being thrown away can provide a valuable datapoint for waste management systems. Students Jacky Wang and Gordonson Yan created their project, called SBTrashCat, to recognize trash types by the sounds they make when being thrown into a bin. Currently, the model can three different kinds, along with background noise and human voices to eliminate false positives. Distributed edge machine learning The abundance of Internet of Things (IoT) devices has meant an explosion of computational power and the amount of data needing to be processed before it can become useful. Because a single low-cost edge device does not possess enough power on its own for some tasks, Jong-Ik Park, Chad Taylor, and Anudeep Bolimera have designed a system where